Luyan Sun’s research group and Dong-Ling Deng’s research group at Tsinghua University have collaborated to carry out an experimental demonstration of training deep quantum neural networks (DQNN) with a six-qubit programmable superconducting processor. The research paper, titled "Deep quantum neural networks on a superconducting processor", has recently been published in Nature Communications.
Over the past decade, machine learning has achieved tremendous success in both commercial applications and scientific research. In particular, deep neural networks, which contain multiple hidden layers and are believed to be more powerful in extracting high-level features from data than traditional methods, play a vital role in cracking some notoriously challenging problems. The deep neural networks can be efficiently trained via the backpropagation (BP) algorithm.
Meanwhile, exciting progress has been made in quantum machine learning field. On the theoretical side, rigorous quantum speedups have been proved in classification models and generative models with complexity-theoretic guarantees. On the experimental side, with the rapid development of quantum devices, some quantum machine learning models, like quantum convolutional neural networks and quantum adversarial learning models, have been successfully realized on quantum devices.
Similar to deep classical neural networks with multiple hidden layers, a DQNN with the layer-by-layer structure is proposed, which can be trained via a quantum analog of the BP algorithm. Under this framework, the quantum analog of a perceptron is a general unitary operator acting on qubits from adjacent layers. However, realizing general unitary operators is still a significant challenge with noisy intermediate-scale quantum (NISQ) devices.
Recently, Luyan Sun’s research group and Dong-Ling Deng’s research group have designed a quantum BP algorithm for DQNNs with the layer-by-layer structure, and demonstrated the trainability and generalization capacities of DQNNs on a superconducting processor. In the framework, qubits are arranged into multiple layers, and the quantum perceptron is defined as a parameterized quantum circuit applied to the corresponding qubit pair at adjacent layers. A sequential combination of the quantum perceptrons constitutes the layerwise operation between adjacent layers. In the forward process, the quantum information is mapped layerwise from the input layer to the output layer. In the backward process, the quantum information is mapped layerwise from the output layer to the input layer. When evaluating the gradients with respect to all parameters at the adjacent two layers, one needs to separately run the DQNN in the forward and backward way, and extract the local information from these two layers, rather than the full DQNN.
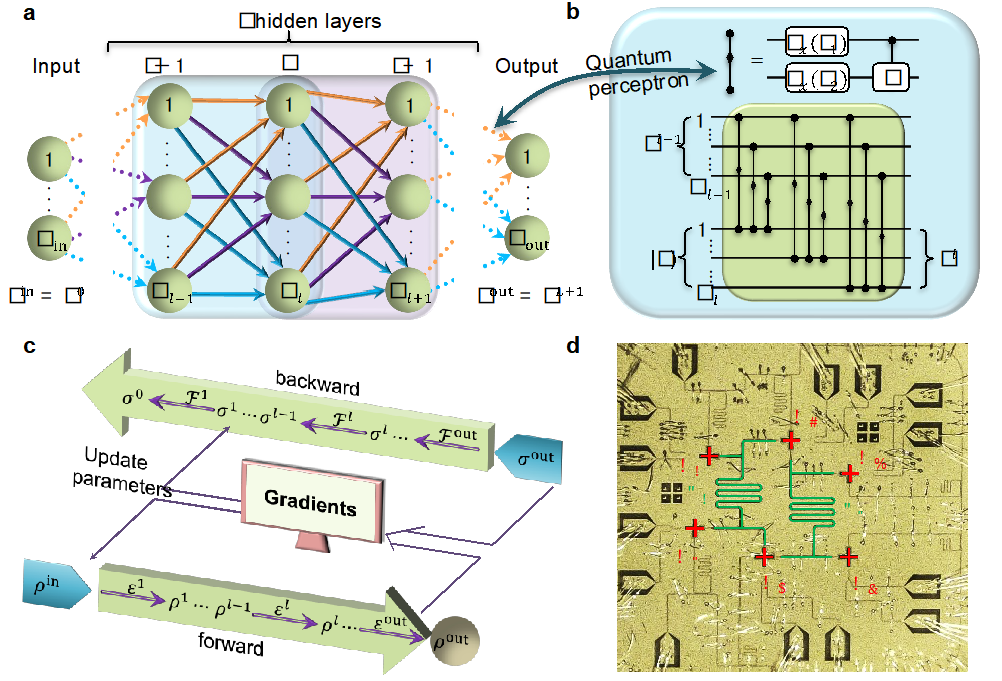
Figure 1. Schematics of deep quantum neural networks and the quantum backpropagation algorithm.
Luyan Sun’s research group and Dong-Ling Deng’s research group have experimentally implemented a three-layer DQNN with two qubits in each layer. The experiment focused on the task of learning a two-qubit quantum channel. The optimization goal was to maximize the mean fidelity between the output state produced by the target quantum channel and the DQNN output state averaged over different input states. In the experiment, the forward process was experimentally performed and the backward process was classically simulated. The quantum states of qubits in each layer were extracted by carrying out quantum state tomography, based on which the gradients were evaluated to update the variational parameters in the DQNN.
The experimental results (shown in Fig.2) indicate DQNN converges quickly during the training process, with the highest fidelity above 96%. With further improvements in experimental conditions, the quantum BP algorithm demonstrated in our experiments can be directly applied to DQNNs with extended widths and depths.
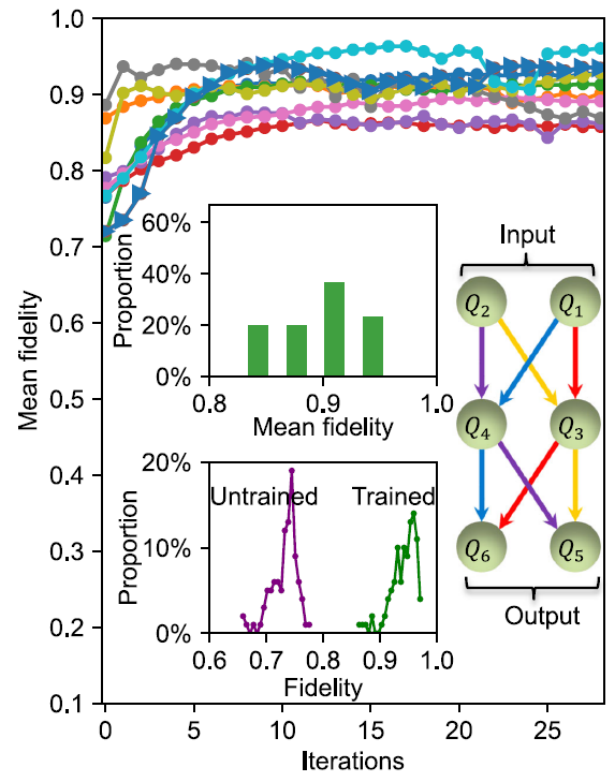
Figure 2. Experimental results for learning a two-qubit quantum channel.
Xiaoxuan Pan and Zhide Lu, Ph.D students of the Institute for Interdisciplinary Information Science, are the co-first authors of the paper. Associate Professor Luyan Sun and Assistant Professor Dong-Ling Deng are the corresponding authors. Other authors include Weiting Wang, Ziyue Hua, Yifang Xu, Weikang Li, Weizhou Cai, Xuegang Li, Haiyan Wang and Yi-Pu Song from Tsinghua University, and Professor Chang-Ling Zou from the University of Science and Technology of China.
Link to the paper: https://www.nature.com/articles/s41467-023-39785-8
Editor: Li Han